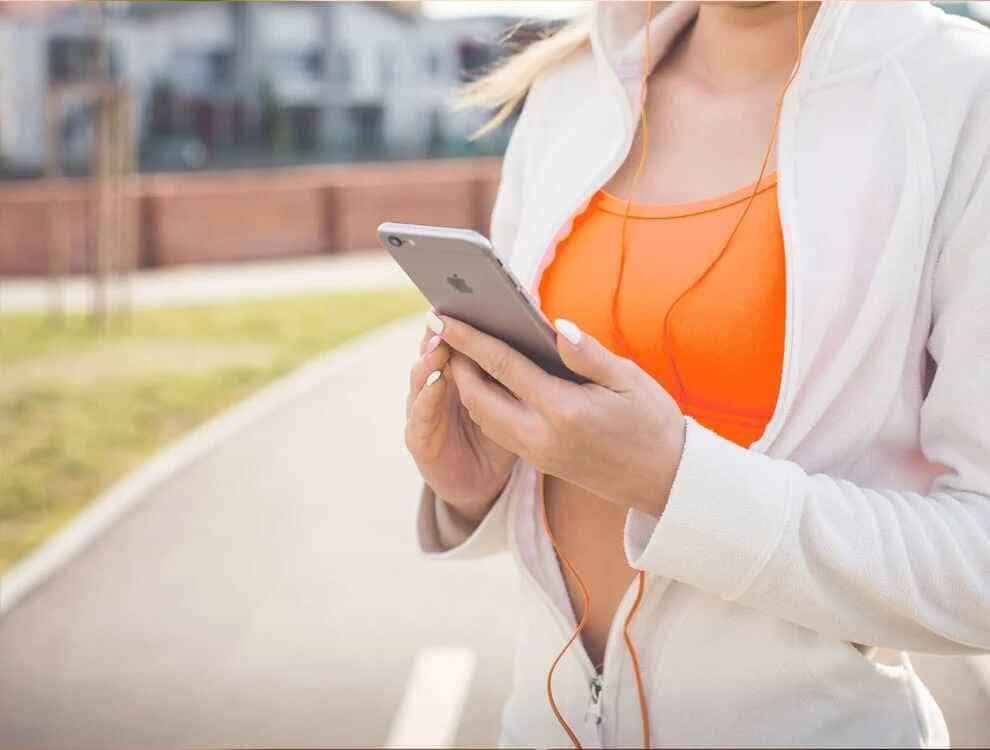
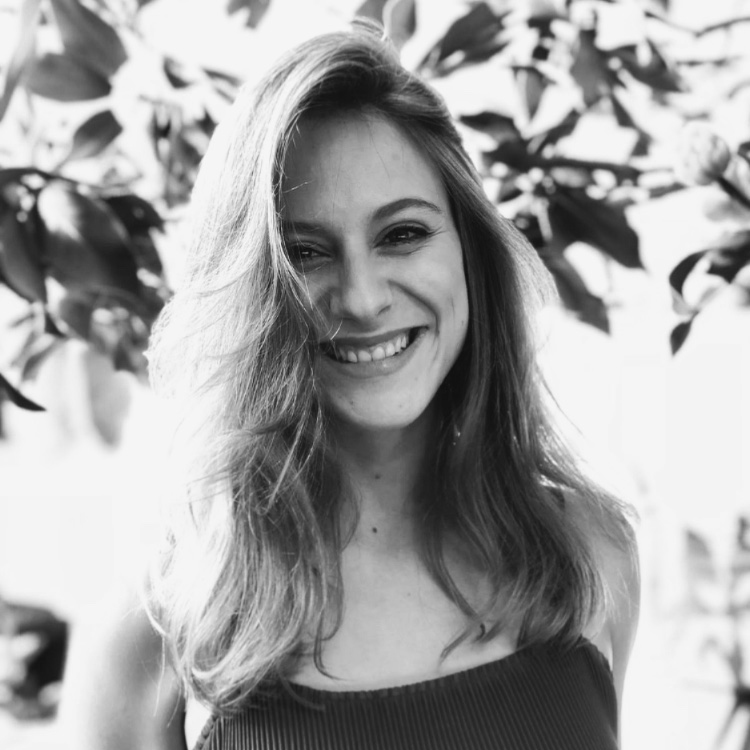
Get monthly notifications
Why does Siri not understand me? AI and language comprehension
One of the challenges that researchers face in Artificial Intelligence (AI) is being able to understand human language and simulate it through technology.
Think of the following situation: you ask for help from Google Assistant, Siri, Alexa or any other artificial assistant and the result you get is far from what you wanted to express. There are times that, depending on the way we speak or the words we write, are very difficult for personal assistants to understand, therefore, it is essential that the AI becomes more skillful when it comes to understanding the human language.
Google has always been concerned with improving that understanding gap in its searches, so today we want to talk to you about how smart systems work through the example of SyntaxNet and its analyzer, Parsey McParseface.
Can you imagine being able to build an application that understands human language? This is the project presented by Google in 2016, which consists of a model of neural networks with open source from which any developer can develop applications linked to natural language.
Wow...! But how does it work?
SyntaxNet with its analyzer, Parsey McParseface, combines automatic learning and search techniques to improve the learning of English and use the language with an accuracy of 94%, according to Google.
For us, humans, speaking is very easy. In fact, we do not think about it, and we only begin to notice that our linguistic system must be quite complicated when we face the task of learning a second or third... languages.
Humans learn to speak from the time they are children, increasing their vocabulary, modifying the meaning and restrictions and intentions of words and adapting the relevance of the words to use in different contexts. We learn from those who are close to us (from the context) and we understand more and more vocabulary (semantics) and structure (syntax) almost effortlessly.
Something similar happens with AI systems, although it is much more difficult to artificially encode all the complexity of human reasoning, since all truths are approximate and encounter many obstacles along the way; for example, the large number of meanings that a word can have or the intention of a sentence in a certain context.
Let's see how SyntaxNet works in the following example:
Given a sentence as input, the processor labels each word with a speech part label, which describes the syntactic function of the word and determines the syntactic relationships between the words in the sentence.
These syntactic relationships are directly related to the meaning of the sentence:
This structure codes that Alice and Bob are nouns and Saw is a verb. The main verb saw is the root of the sentence and Alice is the subject (NSubj) of saw, while Bob is its direct object (DObj).
As you can see, Parsey McParseface analyzes this sentence correctly, but let's see what happens in a more complex example:
Any human can understand this phrase and imagine Alice driving a car down the street. But an AI system may be able to understand what for a human being would be an absurdity: that Alice is driving having the street inside her car. This is one of the main problems that makes the analysis so challenging: human languages show remarkable levels of ambiguity.
Human beings do remarkable work in dealing with ambiguity, almost to the point where the problem is imperceptible. The challenge is creating computers that are capable of doing the same.
SyntaxNet applies neural networks to the problem of ambiguity. Instead of simply taking the first decision at each point, multiple partial hypotheses are maintained at each step, and the hypotheses are only discarded when there are other hypotheses of greater rank under consideration.
The main source of errors at this point are examples such as the previous sentence, which requires knowledge of the real world (for example, that a street is not likely to be located in a car) and also deep contextual reasoning.
Machine learning (and, in particular, neural networks) has made significant progress in resolving these ambiguities; and, while the precision is not perfect, it is certainly high enough to be useful in many applications.
This is just one example of how investigations into AI are trying to improve intelligent systems: understanding the meanings and launching a better hypothesis every time. A great goal, right? And, thinking of how voice Search will affect digital marketing, the challenge increases. Here’s a look at 6 ways voice search will impact digital marketing:
-
Complexity will increase: queries are much complex than they were even 3 years ago due to largely to changes in Search Engine Algorithms that have become less attuned to keywords and more capable of inferring meaning.
-
Interactions will be shorter: people are usually run out of time, this is why quick interactions regarding needs, answers to usual questions… will dominate the communication between humans and assistants.
-
Local search will rise: related to the previous point, shorter interactions are usually used for local things, such as, find the close restaurant, fastest way to go to somewhere… Many voice searches happen on the go.
-
Audio-only interactions will increase: it will be a change in the way of information is presenting. Focusing more on the meaning and the value of the action, rather than images, infographics, and other visual media.
-
Challenge to attain top ranking. Digital assistants only return the top result based on your “voice-search query”, you may not be able to view the lower rankings of possibilities.
-
Conversational content will be important, such as semantics, pragmatics and the impact of content, situations and intentions. It will expect a conversational, human answer in return.
Surely we are closer to the day when it will be very easy to have an informal and natural conversation with our personal assistants. What is not in the slightest doubt, is that with these small-big steps, we have tools at our service, and free access, that bring us closer to an increasingly human Artificial Intelligence.
What about you? How do you live the experience of having conversations with chatbots, personal assistants, answerphones...?
More info: Announcing SyntaxNet: The World’s Most Accurate Parser Goes Open Source
How does Google use natural language processing to reduce the time to insights.
Featured Articles
The Full Funnel Approach and Pinterest
Today, we're going to delve into the significance of working with a full-funnel strategy and understanding why a comprehensive media mix is crucial. We'll also explore an example of how this approach and including Pinterest in the media mix led to a significant decrease in the cost of sales for Houdini.
Master Text Overlays: Boost Ad Engagement
In recent years, capturing the attention of your target audience has become more challenging than ever. Businesses and marketers are constantly on the lookout for innovative ways to stand out in the crowded advertising landscape.
One effective technique to draw attention to your ad's message is through the use of text overlays on image and video ads. In this blog, we will explore the best practices for incorporating text overlays, ensuring your message is impactful without compromising the visual appeal of your ads.
Similar Articles
Protect Your Facebook Business Account from Phishing: Expert Tips by Keywordio
Hello everyone, Johan here from Keywordio. Today, I want to share crucial insights into phishing—what it is and how you can safeguard your Facebook business account from this prevalent threat.
Exploring the Future of TikTok: Insights from the 2024 Trend Report
Introduction
I'm excited to delve into TikTok's comprehensive "What's Next 2024 Trend Report." This report is a treasure trove for anyone in digital marketing, offering deep insights into the future of content, storytelling, and consumer engagement on TikTok.
E-Commerce Excellence: Meta's Advantage+ Shopping Campaigns Redefined
Welcome to the dynamic world of e-commerce, where Meta's Advantage+ shopping is reshaping how businesses connect with customers. In this blog post, we'll explore the key features of this innovative tool.
Introducing TikTok Shop
Today, I bring to your attention a revolutionary update from TikTok, a platform where over 150 million Americans seek daily doses of inspiration and entertainment. With a plethora of trends, fashion hacks, beauty nuggets, and a smattering of recipes, TikTok has emerged as a modern-day oracle for curious minds. The excitement in the air thickens as we unveil TikTok Shop to the US, an avant-garde conduit for folks to spot and snag their adored items seamlessly.
The Full Funnel Approach and Pinterest
Today, we're going to delve into the significance of working with a full-funnel strategy and understanding why a comprehensive media mix is crucial. We'll also explore an example of how this approach and including Pinterest in the media mix led to a significant decrease in the cost of sales for Houdini.
Master Text Overlays: Boost Ad Engagement
In recent years, capturing the attention of your target audience has become more challenging than ever. Businesses and marketers are constantly on the lookout for innovative ways to stand out in the crowded advertising landscape.
One effective technique to draw attention to your ad's message is through the use of text overlays on image and video ads. In this blog, we will explore the best practices for incorporating text overlays, ensuring your message is impactful without compromising the visual appeal of your ads.
The Next Big Thing: 5 Digital Marketing Trends to Watch for in 2023
Digital marketing is a constantly evolving field, shaped by emerging technologies, changing consumer behaviors, and the dynamic nature of the online landscape. Staying on top of the latest trends is crucial for businesses and marketers to effectively engage with their target audience and drive growth. In this introduction, we will explore some of the key digital marketing trends that have emerged in recent times.
The Power of Branding on Meta
Are you ready to unlock unparalleled opportunities and take your online business to new heights? In a rapidly evolving digital landscape, Meta continues to revolutionize the way marketers and entrepreneurs create an impact on buyers.
Today, we will talk about the immense potential of branding on Meta's platforms, Facebook and Instagram based on our Meta Branding (Online Course). Brace yourself for insights that will empower you to establish a strong brand identity, harness consumer trends, and optimize your advertising strategies like never before! Ready to spearhead your business to success? by understanding the potential of branding on Meta and how you can tap into it. Excited? So are we - let's get into it!
A Sneak Peek into Pinterest's 2023 Product Updates!
Pinterest is improving its Ads Manager to provide a seamless experience for advertisers. New features will be released throughout 2023 to optimize advertising strategies. Pinterest aims to offer powerful tools and insights for better campaign results. Get a sneak peek into the exciting updates coming to Ads Manager this year.